All Research Sponsored By:Dataiku
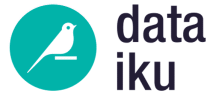
-
3 Keys to a Modern Data Architecture Strategy Fit for Scaling AI
Ultimately, the modern data stack is about providing a seamless experience for all users, no matter what their data needs are. Get 3 key recommendations that will help you determine and build the data architecture that’s right for your teams.
-
The Total Economic Impact™ Of Dataiku
Customer interviews and financial analysis found that a composite organization experienced benefits of $23.5 million over three years and an ROI of 413% with Dataiku. Plus, 80% time savings on manual processes, reduced costs, and improved decision making on key business activities. Get a copy of the full study to learn more.
-
Introducing MLOps
Traditional machine learning operations were fairly simple and easy to manage; but as ML grows in complexity and scope, the old way of doing things is no longer feasible. ML projects are often started in response to C-suite goals and involve employees across the length of an organization. Check out this eBook to learn more about MLOps.
-
A Framework for Choosing the Right Use Cases
How do you know if you AI project is a success? Learn how to define and measure AI success.
-
The State of the Market
Organizations looking to incoroproate machine learning and AI into their large-scale analytics need a certain kind of infrastructure. Learn what enterprise AI platforms bring to the table and how to evaluate them.